How brain chips look through keyholes yet see the full picture
Movement can be thought of as the activation pattern of muscles governed by a series of hierarchical planning steps to reach a certain motor goal. Kalaska (2009) further outlines:
For instance, the most explicit robotics-inspired planning models suggest that the initial stage in planning a reaching movement involves the specification of the final end point, spatial hand path, direction, distance, and speed of the hand from its current position to the target location. In subsequent steps, the motor system determines the pattern of joint rotations to displace the hand along the intended spatial trajectory, then the joint torques necessary to produce those joint rotations, and finally the activation signals to the muscles acting across each joint.
Moreover, this hierarchical cascade of activity (Fig. 11) implicates many brain regions in movement generation, not all of which are easily observable by a data acquisition system suitable for clinical BCIs. From the Posterior Parietal Cortex (”PPC”) encoding the motor intents and linking action with perception (Fogassi and Luppino, 2005) to the spinal “Central Pattern Generator” circuits encoding rhythmic movements of walking (Grillner and Zangger, 1979).

Figure 11; Marel et al., 2019: Review of the neuroanatomical hierarchy. Reprinted from Box 2.
Movements of Daily Living
Most attempts to decode movement consider well-isolated dynamics associated with simple movements, usually following a preparatory phase that unveils the target ahead of the cue to move or speak. Far less explored avenues of Motor BCIs, and more broadly neural population analyses, are non-stereotypical movements. A clinical Motor BCI must be robust to these spontaneous movements of everyday life which may involve factors such as:
Movement goals and initiation are unknown ahead of time,
Movement planning and execution are interrupted or adjusted by environmental feedback,
Complex movement may simultaneously recruit other coupled movements,
Complex movement may simultaneously recruit other independent movements.
Traditionally, the activity of a single or handful of neurons in cortical areas has been found to strongly encode information about movement. This view, however, fails to explain many aspects of behavior (Churchland and Shenoy, 2007), especially when perturbed or complex, preventing any efforts to commercialize Motor BCI decoders that subscribe to this view.
An increasingly popular hypothesis does away with much of the otherwise unexplainable phenomena; it propels Motor BCI research to new heights and spawns a new breed of clinically plausible cortical decoders.
Neural Manifold Hypothesis
In the early 2000s, as the research community adopted technologies to record the activity of many neurons simultaneously, experimental studies started to amass findings that suggest that movement generation is, at least to a large part, the result of the coordinated collective activity of neural populations.

Figure 12; Stevenson, 2015: The number of neurons we can simultaneously record from doubles every 7 years, mimicking Moore’s law. Contemporary data points collected at the Stevenson Lab update the original 2011 figure from Stevenson and Kording (2011).
🍃 To explain this interaction, many support the view that the motor cortex is a dynamical system arranging the activity of millions of neurons (spanning the ”neural space”) into simplified lower dimensional surfaces (”manifolds” embedded in the neural space) on which specific paths (time-varying “trajectories” of latent activity) map to the underlying collective neural activity maintaining the system’s internal state (e.g. avoiding tangling, untuned/movement-independent activity, integrating sensory information), describing its initial state predictive of subsequent activity (e.g. movement preparation), and generating movements (with ”output-potent” trajectories).
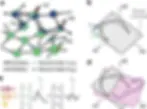
Figure 13; Gallego et al. (2017): “Hypothesis: varied motor behaviors are caused by the flexible activation of combinations of neural modes. a The connectivity of the cortical network results in neural modes whose combined activity explains the specific activity of individual neurons. b The neural space for the three neurons recorded in a. The time-dependent population activity is represented by a trajectory (in black, arrow indicates time direction) mostly confined to a two-dimensional neural manifold (gray plane) spanned by two neural modes (green u1 and blue u2 basis vectors). c The time-dependent activity of each recorded neuron is a weighted combination of the latent activities L1 and L2, each the time-dependent activation of the corresponding neural mode. d Do neural manifolds for different tasks (shown in gray and light purple) have similar orientation? Are the latent activities for the two tasks (shown in black and purple) similar? These are the two critical questions to test our hypothesis.” Reprinted from Figure 1.
Rather than the traditional view of attributing the activity of a few neurons to some external movement variables (as functional somatotopic representations), population analysis techniques reveal that the activity of many neurons is attributed to various internal processes. If disentangled, some of these processes may include movement execution instructions, which can be decoded.
For a pleasant introduction, see Chapter 4 of Pandarinath and Bensmaia (2022). For landmark studies observing manifold dynamics in the motor cortex see Churchland (2012) and, during motor control, Gallego et al. (2017).
To gain intuition of how the brain encodes motor information, imagine a vast landscape with diverse terrains, from rolling hills to towering mountains and serene valleys:
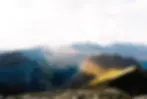
Photo 1; Hosovsky, 2019: View of Kosovo on the way to the peak of Mount Korab, North Macedonia.
This landscape is a lower-dimensional representation of matter and energy that our senses can perceive and our bodies interact with. It is a reduced representation of the outside world our brains construct to satisfy our goals.
Similarly, the neural manifolds of movement reduce our inner world of millions of spiking neurons into a simpler landscape serving our motor goals.
Each manifold represents a class of similar movements — for example, there may be a “reaching” manifold or a “grasping” manifold where individual grips are different trajectories along its surface.
Each ridge or valley in this landscape represents a certain kind of terrain, similar to how each manifold represents a movement class. The high peaks are akin to frequently-used movements that the brain has strongly encoded, while subtle valleys may be more rarely used movements.
As we move through the landscapes, we follow paths and contours at varying heights. Small deviations lead to subtle variations in movement. But larger shifts in neural traversal can bring us to completely different regions of motor space, much like how hiking over a high summit pass can lead us into a new landscape.
Our hiking goals dictate which paths we take. They may alternate between reaching the summit, photographing the landscape, finding shelter, observing wildlife, and so on. As with hiking, only some trajectories on the manifold generate movement, though every movement can be reduced to these trajectories.
The global topology of neural motor space, with its manifold folds, shapes, and interconnectivity resembles the way terrain forms landscapes. Mountains have ranges, foothills, and watershed boundaries; motor manifolds have structure too. Both landscapes and neural representations can be locally smooth but show global complexity across large spaces.
Tracing this inner landscape with movements remains an exciting frontier yet to be fully charted. Still, treating the motor cortex as a dynamical system that encodes movements into low-dimensional manifolds has already yielded substantially improved decoding approaches highlighted in the next section.

Part 5 of a series of unedited excerpts from uCat: Transcend the Limits of Body, Time, and Space by Sam Hosovsky*, Oliver Shetler, Luke Turner, and Cai Kinnaird. First published on Feb 29th, 2024, and licensed under CC BY-NC-SA 4.0.

uCat is a community of entrepreneurs, transhumanists, techno-optimists, and many others who recognize the alignment of the technological frontiers described in this work. Join us!
*Sam was the primary author of this excerpt.