‘Think before you speak’ takes on a new meaning (4/5)
Decoding speech from intracranial neural activity has been considered a dead-end by many researchers. Recently, to their surprise, it rapidly evolved into one of the most promising topics in applied Motor BCI research.
Starting with the foundational work by Kellis et al. (2010), which introduced decoding spoken words from LFPs, the potential for rapid and intuitive communication through direct brain interfaces was recognized. However, the early stages of this research faced significant performance challenges.
Early Progress
Further attempts to harness neural signals for communication, such as the Jarosiewicz et al. (2015) publication on virtual typing by individuals with tetraplegia, showed promise but also highlighted the limitations due to decoder calibration issues caused by signal variability over time. The field saw modest success in phoneme classification by Mugler et al. (2014), who achieved up to 36% accuracy in classifying all American English phonemes using signals from the speech motor cortex, and a slightly better 63% accuracy for single phonemes — figures that underscore the early struggle for precision in decoding efforts.
The pursuit of accurate speech decoding continued with Wilson et al. (2020), who decoded spoken English from intracortical electrode arrays in the dorsal precentral gyrus, and Stavisky et al. (2018), who explored neural dynamics during speech in individuals with paralysis, achieving favorable outcomes despite less-than-ideal recording conditions.
In 2021, Moses et al., (2021) decoded the ECoG data associated with attempts to articulate words by a participant with anarthria into phonemes which, with the help of a natural-language model, were converted into words from a 50-word dictionary.
🗣 Pancho, the participant, became the first person to demonstrate that full words can be decoded from paralyzed individuals, at a modest 15-word-per-minute rate and 75% word accuracy.
The narrative of gradual improvement of decoding phonemes culminated with Duraivel et al. (2022), who nearly doubled the decoding accuracy through high-resolution neural recordings, marking a significant leap forward compared to earlier methods.
This progression, while marked by incremental advancements, also reflects the initial inadequacies in the field — evidenced by the need for alternative methods like handwriting or gesture recognition in early Motor BCIs due to the poor performance of vocal decoders. The journey from the pioneering work of decoding LFPs to the more recent successes in iBCI decoding both highlights the challenges and the potential of Motor BCIs in restoring naturalistic communication, emphasizing the critical role of accuracy and the ongoing quest for improvement in this transformative field.
The year 2023 included several modestly promising papers on the reconstruction of speech from neural data. Duraivel et al. (2023) used high-resolution LCP-TF µECoG electrode arrays to decode speech-to-text with a modest phoneme accuracy of about 50%. Berezutskaya et al. (2023) showcased a classifier that achieved over 90% accuracy, but only among 12 words.
Metzger et al. (2023) presented a neuroprosthesis for speech decoding and avatar control, achieving significant advancements in real-time communication for individuals with severe paralysis. They utilized a 253-channel high-density ECoG array to decode speech-related outputs into text, synthesized speech, and facial-avatar animation. Their system demonstrated accurate and rapid large-vocabulary text decoding, achieving a median rate of 78 words per minute with a 25% median word error rate. Additionally, they showcased intelligible speech synthesis personalized to the participant’s pre-injury voice and controlled virtual orofacial movements for speech and non-speech gestures, all with less than two weeks of training.
The year 2023 showcased a variety of promising decoders, but one project that year stood out as a fundamental breakthrough in the field.
The Breakthrough
Video 7; uCat, 2023: The New Era of Brain Implants — Can you type faster than a completely paralyzed person?
Willett et al. (2023) developed a method to decode speech at accuracies mature enough for the clinical Motor BCI market. What’s more, they decoded Pat’s, the participant with ALS, attempted speech of 62 words per minute (more than 3x the previous record), from a large 125,000-word vocabulary.
Photo 2; Fisch 2023: Pat with the research team.
Using a Gated Recurrent Neural Network in conjunction with an n-gram language model, they decode speech from two Utah Arrays in speech areas of the motor cortex (premotor area 6v). Their most recent baseline model (released after the paper) achieved an accuracy of 91%, nearly matching the industry standard for audio-to-text decoders (95%).
However, their model did have some important limitations. One major drawback was that the representational drift induced by nonstationarities of device-related and neurobiological origin was not addressed directly by the model structure or the objective function. The only step they took to enhance stability was to augment data with noise and time-warp sentences to improve out-of-sample generalization. As a result, their model required daily calibration on a new intake layer to adjust for drift.
🍃 Poor tolerance to neural non-stationarities is a serious flaw for any Motor BCI pipeline intended for clinical use. However, novel nonlinear methods preserving the alignment of neural manifolds have resulted in stable speech decoding (Luo et al., 2023), and robotic arm and hand control (Natraj et al., 2023) without recalibration for months. Although these efforts were tested with ECoG modalities less prone to drift, Karpowicz et al. (2022), Ganjali et al. (2023), and Ma et al., (2023) employed similar principles to iBCI data.
Willett et al. (2023) deployed GRUs in conjunction with the Kaldi language model. Both of these were outdated by the time of publication. A more modern approach could deploy multi-headed attention and leverage Large Language Models (”LLMs”). Moreover, their architecture failed to represent the spatial lattice on which the implant electrodes were arranged, leaving their architecture less sensitive to affine equivariances (small shifts and rotations) that can arise from electrode drift. Additionally, they used a simple n-gram model (3-gram and later 5-gram) in conjunction with Viterbi search to decode and transcribe sentences.
In summary, their model was highly successful, and it is clear how most of the observed shortcomings could be addressed. The Motor BCI-to-text decoder is very near maturity for medical applications. Current-generation models will likely boost brain-to-text BCI performance past the point of viability for clinical use.
Audio Decoders
The exploration of direct brain-to-audio decoders is also underway, but they are yet to mature like brain-to-text models. The direct speech decoder implemented by Anumanchipalli et al., (2019) approximated continuous vocal kinematics of phoneme pronunciation and then decoded these into synthetic speech. The cherry-picked examples shared with their article sound like the speaker has a speech impediment but are mostly intelligible to a careful listener. Angrick et al. (2021) achieved similar results. More recently, Wairagkar et al., 2023 (poster) achieved nearly intelligible decoded speech (see demo) from just 45 minutes of data without ground truth, illustrating both current limitations and the rapid progress as of late.
Moreover, despite these limitations, there are clear avenues toward achieving fast, high-fidelity Motor BCI to audio decoders. Stable diffusion used contrastive learning to align text embeddings with image embeddings, bridging the two domains. Given the availability of high-quality text-to-speech models, it seems feasible to use alignment techniques to bridge the gap between neural data and speech synthesis, thereby leveraging preexisting high-performance models.
Overall, the unexpected emergence of Foundation Models in the domains of text and speech has essentially removed any significant barriers to the full maturation of speech Motor BCIs. Ironically, such complex behavior as speech and language generation may become practical well before robotic Motor BCIs are feasible.
Facial Expressions
In addition to decoding attempted phonemes, Metzger et al. (2023) and Willett et al. (2023) also found strongly encoded representations for other facial movements beyond those involved in speech. Coupling them with speech, allows Users to express themselves further using non-verbal aspects of communication.
Figure 27; Willett et al., 2023: “Confusion matrices from a cross-validated, Gaussian naïve Bayes classifier trained to classify amongst orofacial movements.” Reprinted from Supplementary Figure 3.
Salari et al. (2020) focused explicitly on facial decoding. They utilized electrocorticographic recordings from individuals with refractory epilepsy, classifying five distinct facial expressions based on neural activity. The mean classification accuracy achieved was 72%. Expressions of intended emotion are invaluable for real-world communication, and the end goal of this research is, after all, to restore quality of life to people struggling with motor dysfunction.
Video 8; UCSF, 2023 — “How a Brain Implant and AI Gave a Woman with Paralysis Her Voice Back”. Ann, the participant in Metzger et al. (2023) study, lost her voice to a brainstem stroke over a decade ago. She would like to use her Motor BCI and avatar to counsel others with disabilities.
Integrating facial expression decoding within Motor BCI systems, particularly in VR environments, addresses the critical challenge of personal isolation — a concern accentuated in digital interactions. Much like Apple’s design decision to make users’ eyes visible through the VR goggles of the Apple Vision Pro, the intentional decoding and representation of facial expressions are not strictly necessary for functionality but serve to significantly enhance the social fabric of technology-mediated interactions.
Conveying tone and facial expression are essential for more natural and emotional communication. To do this, we will need to disentangle signals that concurrently encode a variety of facial, articulating, and larynx parameters for speech and expression. More broadly, the problem of integrated decoding or decoding tasks in different domains concurrently is a keystone topic in motor BCIs that has the potential to help bring motor BCIs out of the lab and into the homes of people in need of a comprehensive BCI solution.
Part 9 of a series of unedited excerpts from uCat: Transcend the Limits of Body, Time, and Space by Sam Hosovsky, Oliver Shetler*, Luke Turner, and Cai Kinnaird. First published on Feb 29th, 2024, and licensed under CC BY-NC-SA 4.0.
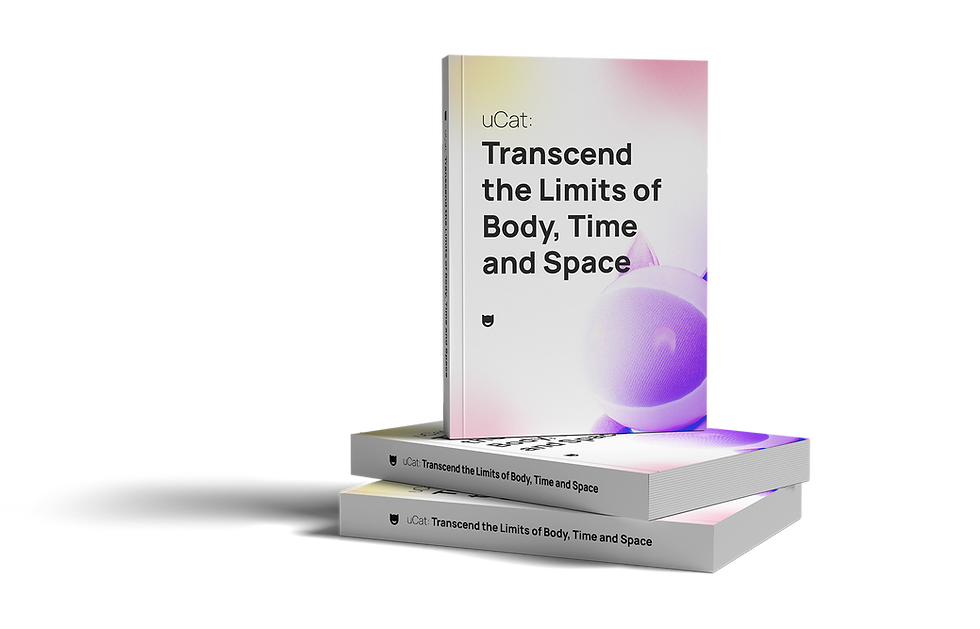
uCat is a community of entrepreneurs, transhumanists, techno-optimists, and many others who recognize the alignment of the technological frontiers described in this work. Join us!
*Oliver was the primary author of this excerpt.
Comments