The challenge of tuning into the right station (5/5)
We have previously discussed three major areas of active research in the Motor BCI field:
Hand and Arm decoding that supports reaching and grasping for everyday mobility,
Finger decoding that supports dexterous tasks, and
Speech and Facial decoding that supports communication and expression.
The last crucial area of research, body part identification (”BPID”), or the recognition of which body parts are being used, has the potential to support the activation and control of Motor BCIs from the other three areas, hinting at the possibility of integrated decoding of the whole body.
In this section, we focus primarily on BPID, with an eye toward the possibility that a BPID model could govern the activation of specialist decoders in an ensemble of expert systems optimized for particular types of movement. We review how Motor BCIs can identify limbs and consider how entangled neural coding affects the prospect of using BPID as a governor for specialist models.
The Compositional Code
Integrated Motor BCIs will require both the capacity to identify active body parts using discrete distinctions made by a BPID model and the capacity to decode complex continuous dynamics for each target body part. While specialized decoders leverage assumptions such as which body part is being moved and what range of tasks are expected, BPID decoders aim to identify the onset of movement in pertinent body parts of subjects who are choosing how to behave endogenously. While this task is ostensibly just simple classification, there is a challenge that renders it non-trivial.
Nason et al. (2021) state the issue clearly:
“It has been noted by several groups that the same neurons can covary with substantially different behaviors […] For example, primary motor cortex can simultaneously encode information about upper extremities, fingers, and speech, independent of body laterality (Cross et al., 2020; Diedrichsen et al., 2013; Heming et al., 2019; Jorge et al., 2020; Stavisky et al., 2019, 2020; Willett et al., 2020). As [decoder scope] increase[s] in complexity, linear models may be unable to discriminate between neural states without sampling greater quantities of relevant neurons.”
Entangled neural coding poses a particularly challenging problem when the goal is to identify the activation of limbs without knowing a priori what limb, much less what task, is being executed. Specialist decoders can work quite well in the lab because — after dimensionality reduction — the chances of entangled coding within a very restricted neural submanifold are limited. By contrast, homeomorphic kinematic dynamics executed across limbs usually share the neural encoding.
Willett et al., (2021a) refer to the sharing of neural codes among homologous kinematics as a “compositional code.” For example, they note that ipsilateral and contralateral wrist movements or same-side wrist and ankle movements share the same trajectory representation (Vid. 10). During unimanual movement, they found a large direction-independent ‘laterality’ dimension coding for the side of the body on which the hand resides. Deo et al. (2024) further validated this observation as their participant simultaneously controlled two computer cursors (Vid. 9) by attempting to move their arms. The laterality dimension was instrumental in helping the RNN distinguish between left and right-hand movements, particularly as neural tuning between the hands became increasingly correlated.
(To play, see footnote link) Video 9; Deo et al., 2024: Two-handed cursor control using an iBCI. Excerpt from Supplementary Video 1.
(To play, see footnote link) Video 10; Willett et al., 2021a: Decoding 32 movement targets across the body.
The term compositional code — as opposed to entangled code — highlights their optimistic perspective, which is born from the observation that, in their dataset, shared trajectory codes are accompanied by a separate code that distinguishes between active limbs. Amazingly, more limb distinctions can improve decoding performance. According to Willett et al. (2021a), “Using more limbs increased the neural separability of targets, enabling more targets to be presented (up to 32 targets at 95% accuracy)” for their ensemble of Naive Bayes Classifiers.
Ordinarily, the performance of a classifier model would decline with the number of distinctions being made by the classifier. However, Willett et al. (2021a) discovered that decoding accuracy improved with the inclusion of more body parts because the movements involved in handwriting are more temporally complex and varied than simple point-to-point motions. This complexity resulted in a larger separation between neural activity patterns for different movements, making distinguishing between them more straightforward for their model.
Unlike typical classifiers that may struggle with more categories due to increased options and potential for confusion, the unique spatiotemporal patterns of neural activity associated with handwriting allowed for better discrimination between different characters and, consequently, higher decoding accuracy (Willett et al., 2021b). Disentangling neural dynamics, as it turns out, can get easier with greater task complexity.
⚠️ A Note of Caution About Brain Region Specialization
A significant hurdle in integrated decoding is the specialization of brain areas, which, while beneficial for focused decoders, limits the efficacy of single-probe implants in capturing comprehensive information. Some brain regions are rich in transferable data, but no single area provides enough breadth for integrated decoding on its own. This challenge is illustrated by Herring et al., 2023, who found robust limb identification performance in areas associated with upper-body movements but less effectiveness in detecting leg and ankle movements. It further underscores the importance of strategic probe placement to adequately capture the necessary information for representing various body parts with high fidelity.
Figure 28; Herring et al., 2023: Decoding performance by limb from a single Utah Array implanted in the Inferior Frontal Gyrus. Reprinted from Figure 5.
Disentangling Contextual Neural Dynamics
To summarize, the complexity of neural codes intertwined with multiple limb movements challenges the efficacy of linear movement trajectory decoding. Yet, a discernible linear subspace encodes body-part activations, making BPID a viable and distinct approach from specialized decoding methods. Moreover, BPID can be enhanced rather than undermined by increasing the number and diversity of body parts to be distinguished. This advancement is promising for developing integrated ensemble Brain-Computer Interfaces (BCIs), although it does come with caution. Models specialized for individual limbs might face interference from the overlapping neural codes of other limbs, intrinsic dynamics, or inputs from other brain regions.
In this context, so-called Latent Variable Models are population models that lead the front in uncovering and interpreting structure from neural population activity. With even a single training example, they can characterize the internal state of biological neural networks based on partial observations of the neuronal population.
The Systems Neural Engineering Lab at Georgia Tech created the Latent Factor Analysis via Dynamical Systems (“LFADS”) method, capable of inferring latent dynamics, underlying firing rates, and external inputs from the activity of large populations of neurons (Pandarinath et al., 2018). They later iterated their method of inferring single-trial dynamics using sequential auto-encoders into AutoLFADS, which enables the training of high-performing LFADS models in an unsupervised fashion for neural datasets of arbitrary size, trial structure, and dynamical complexity (Keshtkaran et al., 2022).
🔑 With access to behaviorally labeled intracranial data across many participants, these Latent Variable Model encoders can define low-dimensional subject-independent dynamics associated with specific movements. These pre-trained models give rise to generalized decoders which are robust to inter-participant differences, and non-stationarities of neurobiological origin, and require only a few training examples to fine-tune for each individual.
While effective at rapidly reducing the high-dimensional neural activity to accurate low-dimensional encodings, the sequential autoencoder inherently uses both past and future data which is unavailable in real-time Motor BCI applications.
Addressing this limitation, the Shanechi lab at the University of Southern California has been at the forefront of pioneering the causal separation of distinct movement patterns from other intricate neural population dynamics.
Their innovative method, Preferential Subspace Identification (”PSID”), has revealed crucial rotational dynamics within neural activities, which are essential for behavioral understanding but often go unnoticed (Sani et al., 2020). PSID has been remarkably revealing, shedding light on the neural basis of various joint movements and thus enriching the predictive value of neural dynamics for behavior.
Enhancing the PSID approach, the integration of an RNN has introduced a more refined modeling of neural dynamics. As detailed in a preprint by Sani et al. (2021), this RNN-based PSID method improves the precision of models related to behaviorally relevant neural dynamics by capturing the inherent nonlinearity, providing a deeper insight into the connections between neural activities and behaviors.
While PSID considers behavior and intrinsic dynamics, it does not account for task instructions passed from other brain regions, like the visual cortex. Vahidi et al. (2024) improved upon this design with IPSID (”I” for input) which can successfully disentangle intrinsic behaviorally relevant neural dynamics from other intrinsic neural dynamics and measured input dynamics.
Furthering these developments, the DFINE method introduced by Abbaspourazad et al. (2023) outperforms previous models by not only enhancing predictions of behavior and neural activity but also by offering a clearer understanding of the underlying neural manifold structures, setting a new standard in the field for complex movement decoding.
In conclusion, the field of motor BCI research stands on the cusp of transformative breakthroughs, driven by a confluence of innovative modeling techniques and a deeper understanding of neural dynamics. Promising solutions increasingly exceed the hurdle that once seemed insurmountable: decoding complex intertwined neural signals. The concept of compositional coding, alongside cutting-edge population modeling approaches such as AutoLFADs, IPSID, and DFINE exemplifies the significant strides being made toward extracting nuanced, behaviorally relevant neural information. These advancements herald a new era of BCIs that can adeptly navigate the intricate neural landscapes of movement and intention, offering precise, responsive control over a broad array of motor functions.
This burgeoning optimism is not unfounded; it is rooted in tangible scientific progress that points toward a future where integrated Motor BCIs can seamlessly merge with daily life, enhancing the User’s independence and QOL. The potential applications of such integrated systems are vast and varied, promising not only to restore lost functions but also to augment human capabilities in unprecedented ways.
As we continue to refine these technologies and overcome the remaining challenges, the vision of fully integrated, highly adaptable BCIs is coming into more explicit focus. With each breakthrough, we move closer to a world where the barriers between thought and action, between intention and movement, are effortlessly bridged by intuitive, empowering technology. The path forward is lit with the promise of greater autonomy and enhanced interaction, opening up new horizons of possibility for all.
Part 10 of a series of unedited excerpts from uCat: Transcend the Limits of Body, Time, and Space by Sam Hosovsky, Oliver Shetler*, Luke Turner, and Cai Kinnaird. First published on Feb 29th, 2024, and licensed under CC BY-NC-SA 4.0.
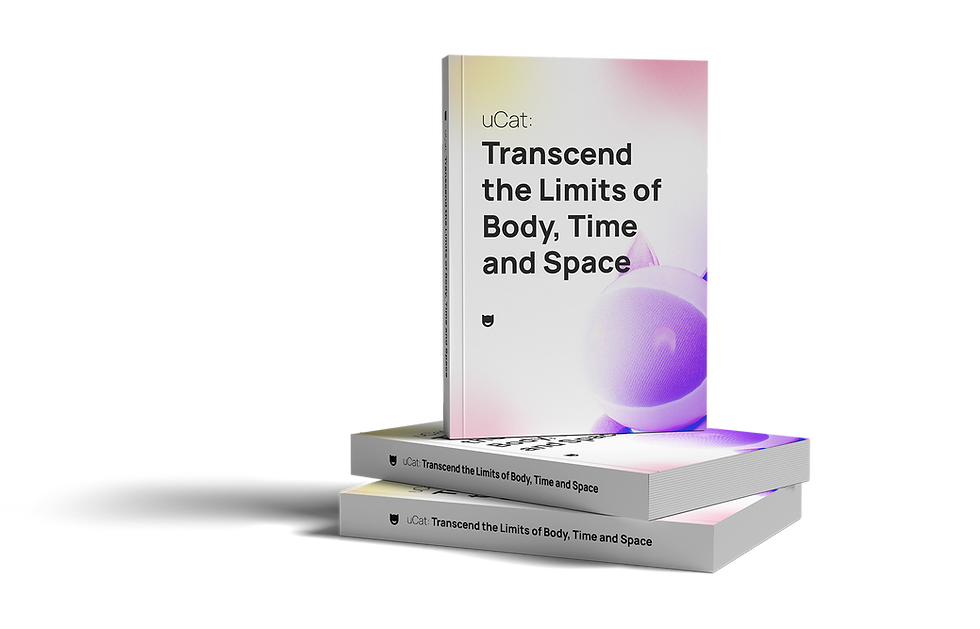
uCat is a community of entrepreneurs, transhumanists, techno-optimists, and many others who recognize the alignment of the technological frontiers described in this work. Join us!
*Oliver was the primary author of this excerpt.
Comments